Artificial intelligence (AI) has progressed significantly in the past few years. It has not only transformed business development but has also shown remarkable breakthroughs in several different industries, such as healthcare, agriculture, and transportation.
A Study at Mckinsey shows that AI/ML models are at the heart of today’s business landscape. These are the tools that help businesses drive profit, revenue, and efficiency. Therefore, the accuracy of AI and ML algorithms is necessary to reap the key business benefits. It’s only possible by overcoming the model development challenges through human intervention in the loop, which is also known as AI+SME in the loop or applied intelligence.
By applied intelligence, businesses can solve AI challenges (discussed later in the article) precisely with Subject Matter Experts (SME). Their expertise, combined with machine intelligence, can help ensure AI model’s accuracy, reliability, and effectiveness. The article will explore the need for AI+SME-in-the-loop for model development and how they can accelerate the process.
What is Human-in-the-Loop Artificial Intelligence?
The term “human-in-the-loop” in artificial intelligence refers to a system that involves or requires human intervention. This approach is used when it is critical to maintaining a high level of accuracy or where the consequences of incorrect decisions could be significant—for instance, medical diagnosis, financial risk assessments, or military operations.
Human-in-the-loop for AI model development requires a person to inspect, validate, or modify some part of the process to train and deploy a model into production.
The Need for Human-in-the-Loop for AI Model Development
Developing an AI model can be complex and require careful planning, attention to detail, and expertise in several areas. AI model development encounters several inevitable challenges under real-world practical situations, which can be alleviated by introducing human intelligence.
Human-in-the-loop can help overcome the following challenges in AI model development.
Data Quality:
Since data is the most crucial asset of the AI system to train the learning model, its quality is critical for the performance of that learning model. Datasets can sometimes be low-quality which can lead to inaccurate analysis and learning.
Heterogeneity and Isolation:
The need for datasets from multiple domains, usually originating from heterogenous and isolated ICT systems, makes it challenging to integrate and process data to build a comprehensive training data set for the learning model.
Limited Labels in Training Data:
Insufficient labels in training data can impact the performance of ML models. Since ML models rely on hand-labeled training data, the cost of building labeled datasets is one of the bottlenecks in ML adoption. Also, the process can be time-consuming.
Feature Construction with Limited Data:
Limited training data makes it challenging to create a high-quality set of input features for your AI model. Feature construction with limited data can lead to inaccuracy in the AI model and the inability to generalize new situations.
Trust & Transparency:
AI systems based on machine learning (ML) are sometimes treated as black boxes, meaning they can make decisions without human supervision. This can be a problem when the task is critical, like in medical treatment or safety control.
Human-in-the-loop and AI Model Development Process
Typically, AI model development consists of three iterative stages: data pre-planning, feature construction and model learning, and model assessment. We can integrate human intelligence into these three stages to accelerate AI model development. Let’s discuss the three phases.
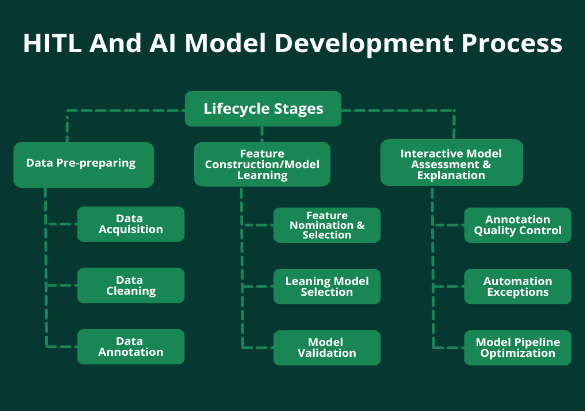
Stage 1: Human-Guided Data Preparation
Human intelligence can be used in data collection and preprocessing procedures to address the challenges of low-quality data and insufficient training datasets. Human computation techniques, such as crowdsourcing, can be used in the data preparation phase, including data labeling, aggregation, cleaning, and integration.
Stage 2: Human-Assisted Feature Construction and Model Learning
Construction of fine-grained features and model learning are challenges for automatic and domain expert-based approaches when training data is limited. Human and machine collaboration is effective in eliciting good features and performing model training. Humans are better at identifying subtle behavioral signals and can assist in feature extraction. Also, they can help compare, select, and steer different machine learning models based on the training data and learning target.
Stage 3: Interactive Model Assessment & Explanation
Adequate trust is necessary to use a machine learning model in the real world, especially for critical systems such as medical, military, and financial systems. Humans involved in the evaluation process can enable quality and robustness assessments and provide model interpretability to bridge the gap between humans and machines.
The table below interprets the three phases and what should be done to achieve successful AI model development. (“What” refers to the problems resolved by each component, “How” refers to the general view in achieving the goal, and “Who” refers to the stakeholders involved in this stage.)
Phases/Stages | WHAT | HOW | WHO |
Human-guided data preparation | Obtaining more labels, clean data, and integrate data. | Crowdsource or demand help from domain experts. | Workers from crowdsourcing platforms, domain experts, or source data managers. |
Human-Assisted Feature Construction and Model Learning | Collaborating with machines to construct feature sets and execute model training. | Design a suitable visualization interface to select/steer models, execute crowd-based data mining over crowd-sourced data, and nominate/select features. | Crowdsourcing workers, AI engineers, or domain experts. |
Interactive Model Assessment & Explanation | Quality assurance, presenting reasons behind the AI models’ decisions. | Design human-computer interface, visualization, and adopt explainable models. | AI engineers or domain experts. |
How Human-in-the-Loop Benefits AI Model Development?
Today, the majority of AI projects fail due to poor data quality, labeling, or modeling. Deploying humans in different stages of the AI model development process can improve models and their outcomes. Benefits of human-in-the-loop for AI model development include.
- Continuous improvement of machine learning and deep learning models through data labeling, quality control, and modeling.
- Human-in-the-loop evaluates the value of raw sourced data to yield more accurate results in the AI model.
- They help in annotating (labeling) source data accurately.
- They understand the logical reasoning behind a process and can design the AI model with specific outcomes in mind.
- More efficient than fully automated approaches, particularly when the amount of data being processed is large.
Practical Application of Human-in-the-loop AI
The main goal of the HITL approach is to achieve more accurate results in a shorter training time. It can play a vital role in training self-driving cars. Self-driving cars can drive well but at times only with 90% accuracy. Since traffic signs may vary in size, color, and text depending on the country or region, the algorithms in autonomous cars may find it hard to label. Here, human-in-the-loop can help label data properly and ensure accuracy in different scenarios.
For example, they can help to improve the safety of self-driving cars by providing a mechanism for humans to intervene in the decision-making process of the AI, especially in situations where the AI may be uncertain, or the consequences of a decision could be severe.
AI+SME in the Loop: Solution to Your AI Challenges
Subject matter experts help ensure the quality of the AI model and are critical in its testing phase. They are responsible for optimizing the performance of AI models and troubleshooting any issues that may arise during the development process. Aisme Labs hosts a dedicated team of SMEs with extensive knowledge in AI model development. They can help you plan your AI strategy and build effective AI models. Get in touch to hire SME for your next AI project.